Most people think modeling is the hardest part of artificial intelligence.
But really, the most obstinate AI barrier isn’t code or sampling, according to Ryan Kraus, Staff Solutions Architect for leading enterprise open source provider Red Hat; instead, it’s having user-friendly infrastructure available to consistently develop AI.
“Right now, they need to focus on building that infrastructure in a way that isn’t going to limit them in the future, which is a pretty tough ask,” Kraus said.
So, where does that leave agencies, many that have spent years accumulating static hardware? Well, they need to move away from the monoliths of the traditional data center model, said Jeff Winterich, Defense Department Account Team Technologist for Hewlett Packard Enterprise (HPE), an edge platform provider.
GovLoop recently interviewed Kraus and Winterich about how agencies can develop and scale AI across the enterprise. They offered the following steps.
1. Be ready to move away from monolithic data centers.
Legacy computing structures always glued data scientists to data centers. The two were tethered together, meaning scientists couldn’t work where the data didn’t reside, much like how a lab scientist needs their lab chemicals and instruments.
Data science, however, is not entirely like lab science, because endless inputs come outside of a controlled environment. AI models are most effective when exposed to open air.
“We have accelerators on our phones. We have compute everywhere,” Winterich said.
The solution is to bring software-based applications to the edge, except for massive data projects.
Software is available anywhere – it just has to be downloaded onto hardware – and can receive updates over the internet, so agencies aren’t weighed down by having to send information to the cloud or data center for processing.
2. Embrace containers and open source.
The most portable, standard software unit is a container. Containers are self-sufficient packages of code that contain all that an application needs to run.
Since containers include all dependencies, they are well-suited to environments everywhere. As long as containers are orchestrated and standardized, they can intake, process and execute data consistently.
3. Bring AI to the edge.
Now that containers are on the edge, AI can join them. Agencies presumably already have devices with compute at the edge, but those often can’t tap into the rest of the enterprise.
The key for agencies is to roll out all the security, storage and processing power they need on the edge. With containers as a standard unit of exchange, the data doesn’t have to travel back to the cloud or data center. Instead, AI can analyze it on the edge, where it’s generated.
Red Hat, HPE and NVIDIA — an AI specialist — have partnered to develop such a solution. KubeFrame for AI-Edge is a turnkey, field-deployable solution that combines the three vendors’ offerings into an out-of-the-box, open source-based AI system.
Processing data at the edge can mean faster analysis and better models.
“The value we’re talking about is making data science easier,” Winterich said.
This article is an excerpt from GovLoop’s recent guide, “The State of AI in Government: Policies, Challenges & Practical Use Cases.” Download the full guide here.
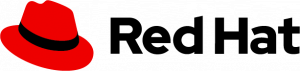
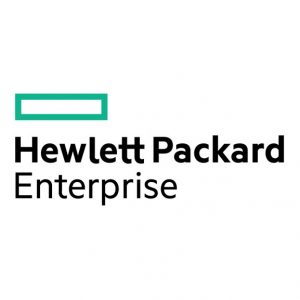
Leave a Reply
You must be logged in to post a comment.