Every vendor that engages with government agencies is likely touting some type of generative AI (GenAI) or Large Language Model (LLM) solution in an effort to capitalize on the considerable attention generated by these technologies over the last 11 months. These AI systems claim to support a number of key capabilities such as natural language generation, translation, and text summarization.
Carefully prepared demos appear to address all kinds of existing customer support issues, and most offerings appear to be the same. So, how can government agencies cut through the similar-sounding product narratives to understand and evaluate the solutions that would work best in their environment? Before jumping in, government agencies should start by debunking certain misconceptions:
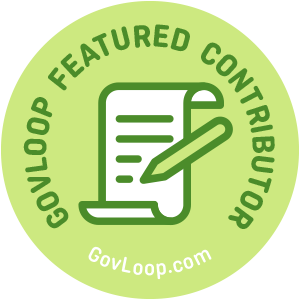
Myth #1: Adding GenAI to the existing solution will yield better outcome
While GenAI can provide valuable assistance, it cannot miraculously solve foundational issues related to volumes of information and relevance of searches through that data. If the existing tools are not able to reliably surface relevant information immediately, bolting GenAI onto these offerings is not going to make them work better. Similarly, if a solution did not effectively answer questions in the past, the mere addition of GenAI is not going to change its performance. Put simply, when it comes to GenAI, garbage in produces garbage out.
Myth #2: GenAI coupled with RAG will end AI hallucination
Both government agencies and vendors clearly understand the limitations of GenAI with respect to hallucinations — which is when the output produced by the model is either nonsensical or outright false. The current buzzword that vendors are promoting to avoid this is called Retrieval Augmented Generation (RAG), which is an AI framework that attempts to provide narrow and relevant set of inputs to GenAI to yield an accurate and reliable summary.
However, the execution of this task is not simple, and consequently not all instances of RAG are created equal. For instance, if RAG yields pages of results that may or may not be accurate and defers the task of deciphering the correct answer to GenAI, the outcome will once again be subpar and unsuitable for agency use. GenAI faces the same challenge as a human would in trying to summarize 10 pages of relevant and irrelevant data. In contrast, both GenAI and humans do a much better job synthesizing 10 relevant sentences.
It’s imperative for organizations to meticulously assess the history of consistently providing accurate and reliable results with RAG-enabled tools. To do so, companies should evaluate the tool’s competence in comprehending context, handling intricate queries, and adapting to changes in language or industry terminology.
This is because the quality of any response generated by the LLM hinges on the quality and brevity of the input documents — with shorter, more concise, and more relevant inputs yielding the best results. Additionally, organizations should test the tool using their own data and introduce changes on the fly, rather than letting the vendor spend weeks retraining and fine-tuning the system to see how it responds to questions related to your specific business.
Successful GenAI Depends on a Solid Data Diet
While generative AI and LLMs have made substantial strides in language understanding and generation, they do not offer a quick fix for the challenges associated with sifting through large volumes of information and providing accurate summaries.
These tools also must be fed accurate and reliable information. As a result, AI’s effectiveness in this context is entirely dependent on the quality of the initial search as the input to the GenAI. To truly make sense of the generative AI hype, it’s essential to address the technology’s limitations and use AI as a powerful tool within a broader information retrieval and summarization strategy.
Ryan Welsh is the founder and CEO of Kyndi, a global provider of the Kyndi Platform for the Natural-Language-Enabled Enterprise, an AI-powered platform that empowers people to do their most meaningful work. To learn more visit https://kyndi.com/ or follow them on LinkedIn and Twitter.
Leave a Reply
You must be logged in to post a comment.