
Federal HR professionals with access to electronic employee databases typically have access to a wealth of so-called “structured” employee data, or information that can be quickly counted and analyzed in spreadsheet programs to create pivot tables and reports. Examples of structured HR data include employee salary and demographic information and employee survey responses, all of which are powerful tools to analyze and predict employee turnover and understand employee opinions.
What about “unstructured” data?
Another less known, less easily analyzed, but potentially potent source of predictive knowledge is so-called “unstructured” or “raw” HR data. Unstructured data is information that is much harder to count and organize in spreadsheets, but can potentially yield powerful results if used in conjunction with text analytics. Examples of unstructured data include stories or essays, narratives in employee performance evaluations or Individual Development Plans, written answers to free response questions, or employee reviews of the agency on websites such as Glassdoor.com.
Text Analytics Software
Today, a wide variety of software programs are available to analyze unstructured text data. Analysis of unstructured data typically involves a focus on words and phrases that appear most frequently, categorization of the observed words into associated groups, and an analysis of the context of words and adjectives that are used in the data, in order to better clarify employee opinions or “sentiment” on certain topics.
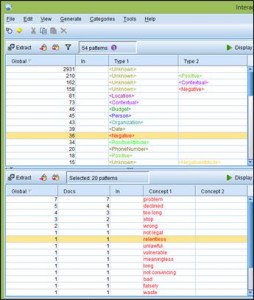
In this SPSS Modeler screenshot from an assignment I completed in graduate school, text is analyzed from public press releases issued by the American Civil Liberties Union via RSS feeds. SPSS recognizes and categorizes the raw text data to identify and count positive and negative ideas and words. The number of times certain phrases appear is also listed. Text analytics software can identify and organize concepts in otherwise unstructured text data.
The software also relies on pre-defined dictionaries to categorize concepts (i.e., “insurance” and “time off” may be categorized under “benefits” using an HR text analytics dictionary). An example of a text analytics program that I personally used in graduate school is IBM SPSS Modeler with Text Analytics. The text analytics add-on to Modeler enables users to input text data from a variety of sources (including documents and RSS feeds), and depending on the resource dictionary used, categorize the data into repeatedly observed concepts or categories, and identify positive or negative opinions. SPSS Text Analytics has several text dictionaries available to interpret the text data, including an employee satisfaction resource dictionary, that recognizes and analyzes concepts and topics that frequently appear in HR.
How can text analytics be used with structured data in the federal government?
Surveys
Structured Data: Surveys that ask “yes or no” questions, or Likert scale survey questions that ask employees to rank responses from positive to negative (such as the Federal Employee Viewpoint Survey), enable HR professionals to quickly count the number of positive or negative responses and sort by demographics, job and geography. However, Likert scale and yes/no questions have some limitations, such as pre-defining answers to certain inflexible categories.
Unstructured Data: Survey questions that begin with “why” and “how,” and allow respondents to provide character-limited free responses, can allow employees to more creatively provide feedback and create a source of text data to identify positive and negative sentiment. An analysis of free response questions can reveal frequently repeated phrases or concepts that might not otherwise have been known to management when the survey was designed. Text analytics can also identify which employees are most likely to express positive or negative sentiment.
Individual Development Plans (IDPs) – Frequently used by federal agencies to enable employees to list short- and long-term career development goals and training associated with goals.
Structured Data: Portions of IDPs that have pre-defined checklists, such as employee demographic data including name and grade, as well as the number of employees with IDPs in place, can provide a source of structured data. An analysis of structured IDP data could reveal which parts of the organization have employees who are most likely to have created an IDP, and if having an IDP is correlated with career success or turnover.
Unstructured Data: Employees’ narrative descriptions of their goals can indicate if certain concepts are frequently repeated in the goal descriptions, which can help management identify training needs for the organization.
Performance Evaluations
Structured Data: The overall numerical rating for the employee is the most evident source of structured data in federal employee performance evaluations. Agencies may seek to evaluate the extent to which highly rated employees are promoted, or leave the agency.
Unstructured Data: The narratives often included in federal employee performance evaluations can be analyzed to identify concepts, and linked to the numerical rating to determine if certain concepts are correlated with a higher performance rating.
Recruiting
Structured Data: Responses to multiple choice questions on applications such as USA Staffing can help determine if an applicant is qualified for a position.
Unstructured Data: Text that applicants place into resumes can be scanned and matched to a job announcement to help evaluate and potential hire’s qualifications for a position. Recruiters can also mine through resumes searching for university majors, GPAs and specializations.
Text analytics can save time!
Text analytics capability can prove extremely useful to HR by reducing the amount of time needed to sort through voluminous unstructured employee data. Instead of manually picking out concepts that are frequently repeated in employee surveys from thousands of employees, text analytics software can automatically and accurately list common concepts, as well as determine if they reveal positive or negative sentiment. Text analytics can prove to be a powerful tool for agencies seeking to derive more meaning from their data.
Matthew Albucher is part of the GovLoop Featured Blogger program, where we feature blog posts by government voices from all across the country (and world!). To see more Featured Blogger posts, click here.
Another great article on how text analytics can be apply to human resources: http://provalisresearch.com/blog/human-resources-text-analytics/
This blog post too shows how text analytics can identify key drivers in HR data. You can request the report as well http://odintext.com/blog/hr-survey-lie-get-truth/