By: Winston Chang, CTO, Global Public Sector, Snowflake and Wolf Ruzicka, Chairman, EastBanc Technologies
In the realm of enterprise technology, the adoption of artificial intelligence (AI) is often driven more by tactical objectives than by strategic foresight. And yet there are many examples of organizations that have become “data-driven” and “AI-enabled,” creating significant value from this trait for their customers and themselves.
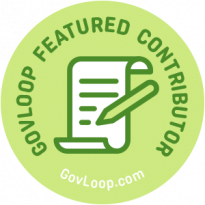
So, what transforms an organization from making tactical AI choices to strategic ones?
Demystifying the Problem in AI
One measure of success is how well AI-enabled enterprises understand their use of AI for long-term success. While “AI” can often seem abstract and complex, it can be framed more simply as the ability to make predictions based on complex data processing. To deliver these predictions, AI initiatives leverage four main resources: compute power, mathematical models, data, and the problem definition.
Removing Roadblocks to Alignment
Adopting AI in enterprises comes with a host of challenges that can impede progress and impact success. One of the primary obstacles is data quality and readiness. Do we have sufficient complete, consistent and current data to solve the problem? In addition, there is often a lack of alignment between AI initiatives and strategic business goals, resulting in projects that are technically sound but fail to demonstrate value. And finally, integrating useful AI into existing workflows requires changes in the organization’s processes and culture. Cultural disconnect can create resistance after a solution is delivered.
Adopting the AI Factory Model
To improve the outcome of AI initiatives in an enterprise, the AI factory model offers a structured approach to creating alignment. The factory orchestrates the creation of the problem statement, with agile development using the data, resources and models available to the enterprise. This resembles the software factory model, which provides a unified concept to create bespoke, high-quality enterprise software solutions while acting as a catalyst for culture change.
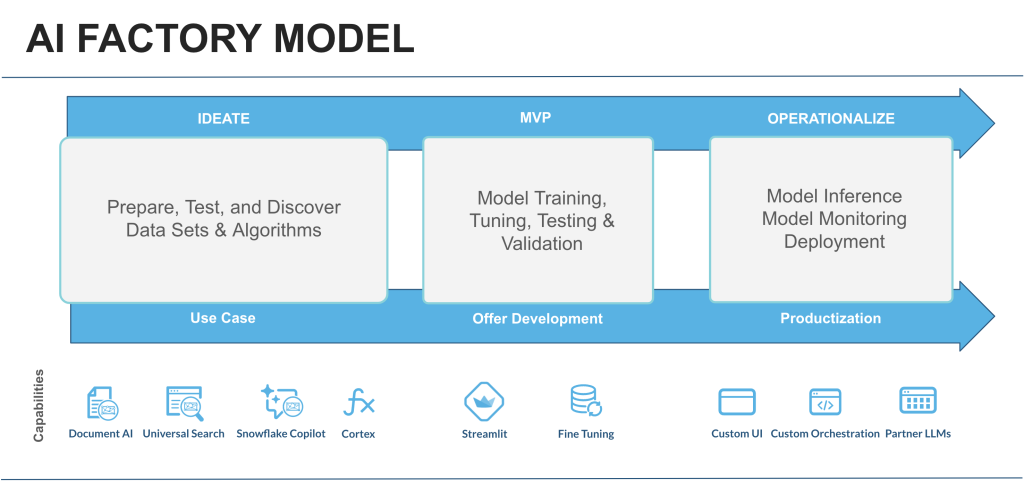
Here’s a high-level overview of how the AI factory process works:
- Ideate: This phase is all about rapid prototyping to discover usable data sets. Start by developing the problem statement. Next, test various data sets and algorithms by developing prototypes and evaluating their functionality to support AI. Additional data sources and cutting-edge algorithms from the market can then be used to refine and enhance your AI solutions.
- Enact MVP: In the minimum viable product (MVP) phase, compute, algorithms and data become runtime use cases. Using those data sets found in the ideate phase, you can train algorithms to learn patterns and make predictions. Tuning involves adjusting hyperparameters and model settings to optimize performance, while testing evaluates the model’s accuracy and generalizability on unseen data. Validation ensures that the MVP meets essential requirements and can handle real-world scenarios before scaling up.
- Operationalize: In this phase, model inference involves integrating the trained AI model into production systems to make real-time predictions or decisions. Model monitoring continuously tracks the model’s performance and accuracy to ensure it remains effective and responsive to changing data. Deployment entails scaling the AI solution across the organization, and ensuring it is fully operational and seamlessly integrated into existing workflows and processes.
Effective AI initiatives require meaningful coordination across many operational functions to create impact. If designed cross-functionally, the AI factory creates an openness for AI adoption.
Tips for Successful AI Adoption
- Start small: Begin with projects that offer quick wins and have clear, measurable outcomes. This helps build confidence with and demonstrate the value of AI to stakeholders.
- Focus on data quality: Ensure that your data is clean, complete and timely. High-quality data is essential for successful AI outcomes.
- Iterate and improve: Adopt an iterative approach to AI deployment. Use initial projects as learning opportunities, then continually refine your processes based on feedback and results.
- Foster collaboration: Encourage collaboration across different departments, including IT, data science and business units. Successful AI projects often require input from multiple stakeholders.
- Ask creatively: Often real-world data and algorithms will not reach levels of accuracy (or fit) that seem usable. But if the organization redesigns the hypothesis, it can become very functional. An example might be a 70% accuracy rate predicting which customers will leave. Altering the hypothesis to look at who will stay might yield a 99% accuracy rate for those it predicts will stay.
In this series we are presenting the AI factory model as a rapid development process that supports AI adoption and culture change for public-sector enterprises. In the posts that follow, we will cover each of the phases in more detail, and wrap up the series with a post on culture change.
Leave a Reply
You must be logged in to post a comment.