Outdated mainframe systems pose significant challenges to innovation and customer satisfaction. These systems, built for a different time, struggle to meet the demands of today’s businesses. By applying machine learning (ML), organizations can reduce customer friction and generate ML use cases that empower teams to create deeply user-centered products.
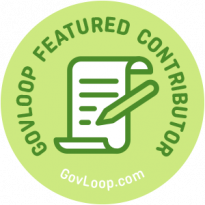
Reducing Customer Friction and Enhancing Experience
Customer friction refers to the obstacles and challenges that users encounter when interacting with a system. In the context of legacy mainframes, this friction often arises from outdated user interfaces, cumbersome processes, and business rules that are based on typical user scenarios that fail to be adaptive to the varied lived experiences of users. The lived experience reflects the overall satisfaction and perception of users as they navigate these systems.
Friction manifests in slow response times, complex processes, and interfaces that no longer meet user expectations. ML plays a crucial role in making systems adaptive to user context, enabling a smoother adoption of modernized systems while improving the overall user experience. The first step in reducing customer friction is understanding where it occurs.
ML excels at analyzing large volumes of interaction data to identify specific points where users encounter difficulties. For instance, machine learning algorithms can see patterns in user effort and behavior in different types of logs, such as transaction logs, SMF logs, JES Logs, IMS, etc., to pinpoint where delays happen most frequently, whether in processing times, number of round trips, navigation difficulties, or repeated completion errors. By mapping these friction points, organizations can prioritize the most significant issues. This targeted approach ensures that the modernization process addresses the areas causing the most frustration, leading to a more efficient and user-friendly system.
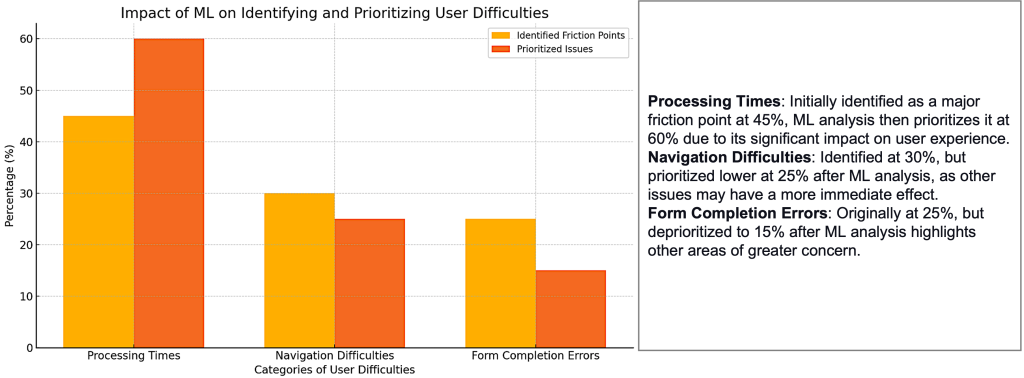
After identifying the friction point, agencies can employ ML to provide specific, actionable insights and use generative AI (Gen AI) to create new possibilities for redesigning workflows and processes. Repetitive tasks that previously slowed down user interactions are automated. For example, in legacy systems where customer information was manually captured across multiple forms, agencies can employ machine learning to automate this input by training models on historical data, such as transaction records, system logs, user interaction logs, and data from databases. This approach allows the system to recognize and replicate patterns of data entry across various forms.
Patterns recognized by machine learning allow fields to be pre-populated by analyzing large datasets to identify commonalities in user inputs. Machine learning models are further trained on these patterns, enabling the system to predict and fill in required information across forms. This significantly speeds up interactions by reducing the manual input required from users.
User behavior is analyzed by machine learning to optimize complex workflows. This is done by collecting and processing data on how users interact with the system, such as the steps they take to complete tasks or the screens they frequently visit. Machine learning algorithms then identify inefficiencies or bottlenecks in these workflows and suggest more intuitive paths. For instance, if users frequently navigate through multiple screens to complete a task, the system might recommend consolidating these steps based on learned patterns, creating a single, streamlined interface. This approach not only reduces cognitive load but also shortens task completion times, ultimately enhancing overall user satisfaction.
Enhancing ML Use-Case Generation for BAs and Product Owners With GenAI Tools
Business Analysts (BAs) and Product Owners (POs) play a crucial role in bridging the gap between business needs and technology solutions. While they bring valuable expertise in understanding business processes and user needs, they may not always have the specialized skills needed to explore the potential of ML fully. The introduction of GenAI toolkits, such as AWS Bedrock, Azure OpenAi, and ReDuX (GoRedux.ai), enhances this capability by transforming how BAs and POs generate ML use cases.
GenAI tools complement additional capabilities by analyzing business rules and database schemas, providing innovative ML use-case suggestions that they can then explore and refine. This cognitive collaboration enables BAs and POs to unlock new possibilities, creating more adaptive and user-centric solutions that align with both business objectives and user needs. Besides, these GenAI tools can be prompted to take a deeply user-centric approach, focusing on personalized contexts rather than rigid business rules.
For example, a Fleet system today can have a standard rule of replacing vehicles at 50,000-60,000 miles, and BAs and POs can use AI to generate ideas using all available data that represent users’ behavior — such as driving history, telematic, and vehicle performance and to suggest useful ML use cases that optimize vehicle replacement timing. Moreover, GenAI can also enable the evaluation of the feasibility and viability of these ML use cases by simulating scenarios and assessing their impact on business operations and user experience. We ensure technical soundness by validating solutions against existing systems and infrastructure practical for real-world applications.
To conclude, it’s important to remember that a one-size-fits-all approach may not be suitable for ML models. These models are designed to adapt and tailor their recommendations to the unique characteristics of each legacy system and the specific non-functional requirements of the target system, providing you with the reassurance of their flexibility and adaptability.
Kartik Mecheri is the Chief Architect at Karsun Solutions. A dynamic leader with a rich background in technology and management, Kartik has spearheaded large-scale digital transformation projects while keeping Karsun’s customers at the forefront of technological advancements. His expertise in cloud computing, modern software development, and enterprise modernization ensure Karsun’s customers receive cutting-edge services while enhancing their internal processes. That includes integrating emerging technologies such as artificial intelligence and machine learning with current modernization practices. The latest release from the Karsun Innovation Center is ReDuX AI, a toolkit that addresses the challenges associated with the migration of complex legacy systems.
Leave a Reply
You must be logged in to post a comment.