By: Winston Chang, CTO, Global Public Sector, Snowflake, and Wolf Ruzicka, Chairman, EastBanc Technologies
The Chicken or the AI
There is a debate about data readiness: Does an organization build artificial intelligence (AI)/machine learning (ML) solutions based on the data it stores, or does it start by implementing business imperatives, and then identify data in its stores to feed those solutions?
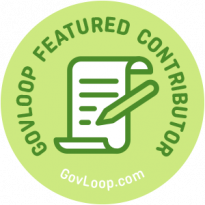
In an AI Factory you do both. Public-sector data collections were historically created for descriptive statistical and light analysis, often leaving gaps in data readiness when it comes to AI/ML. Some of these gaps will require different collection strategies and others can be addressed by the open data market. And because enterprises are just now integrating AI/ML into their workflows, there is room for informing business priorities alongside designing for new capabilities.
Factory Ideation
To get this cyclical process started, the AI Factory hosts a half-day ideation session with an organization’s key “impact” stakeholders, ranging from the CTO or CIO to line-of-business leads to data analytics practitioners and other key functional representatives.
During this workshop, we identify existing AI opportunities and rank them in terms of business value and AI readiness. Opportunities could range from analytics and reporting to new products and process automation. Some questions to ask at this stage are:
- Which processes are automated, semi-manual or manual?
- How well are processes documented, and how complex are they?
- What methods can we use to best calculate mission, resource and budgetary impact?
- Where are there overlaps with the current and future needs of the organization?
- Which resources are currently available for projects?
- Which resources tied up with low-impact work might be freed up?
- How do we assess the availability and accessibility of good data?
We then visualize all of this through an operational heat map. This tool allows us to prioritize opportunities by ranking them according to AI and data readiness across anticipated business value.
The Big Question: Are We Data-Ready?
With potential projects identified, the next step is to double-check data readiness for any use cases on the priority list. The foremost priorities are assessing data cleanliness, data completeness and existing data flows.
The second area to evaluate at this stage is which external sources of data are needed to fill gaps or augment the enterprise’s captive data. Often the value of data goes up when combined with other data. This is especially true for public-sector data.
The final check is to identify the viability of these data sources. Today’s technology makes testing data in AI/ML algorithms fast and insightful. Running the data through AI/ML functions and looking at explainability can provide critical data points on the data’s readiness for AI. Also, the act of validating data readiness assumptions naturally feeds back into your enterprise’s project priority ranking for each use case.
Data Meets Operations
Because the AI Factory is intended to help organizations become AI-enabled, it’s useful to pause to look at the bigger picture. Data is the “currency” driving the two components of a successful AI solution: compute and math (models). The right type of data (as determined by data science) in the right format, volume and flow (as created by data engineering) is essential for model training and continuous execution. So, every successful AI Factory needs a disciplined way to source and manage its data flows.
To this end, two important processes must be added to the AI Factory’s enterprise’s development cycle: data operations (DataOps) and AI/ML operations. DataOps provides the methodology that prevents data chaos, and AI/ML operations provides an observability system to manage cost, quality, risk and efficacy. DataOps also provides processes and tools to make new data sources ready for use — so that AI/ML projects can iterate, learn and mature.
Beyond extending the “factory floor” of the software factory concept, the AI Factory model introduces a set of reinforcing processes — from iteration to operations — to ensure that its products (e.g. AI/ML solutions) are able to continuously improve after they are released into production — and ultimately into high-value workflows.
Seeking Possibilities
While rapid development is an increasing capability of many organizations, innovation is still driven by prioritization. Resources are never unlimited. The point of the ideation phase is to allow for fast exploration of possibilities within a methodology prioritized by the right leadership. We offer one of many ways in which this can be achieved.
The ideation phase ideally draws out creativity from key members of the enterprise and aligns expectations for subsequent development (e.g., through proofs of concept, minimum viable product assessments and production-deployment analyses). And, if done right, it begins the essential change management process.
Read Part One of our AI Factory blog series here.
Leave a Reply
You must be logged in to post a comment.