With all of the hype and abundance of options for generative artificial intelligence (GenAI) solutions, the allure of building your own solution can be irresistible, especially for technically proficient government agency teams. With the availability of various and inexpensive elements such as vector databases, deployment frameworks, and large language models (LLMs) from multiple sources, the process may seem straightforward at first glance.
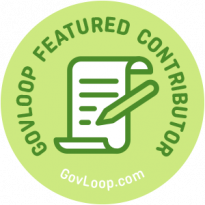
However, in reality, it is much more involved. One of the initial pitfalls of building your own GenAI solution is the deceptive nature of the initial results. Technical teams can easily download different components, stitch them together, and construct a seemingly functional solution that gets them 60-70% of the way there immediately. It may seem that merely by working harder, the technical team can bridge that last 30-40% gap, but after months of effort, the solution is only a few percent better and still does not meet the needs of the agency. Perhaps with just a little more dedicated effort, and then it will work well?
The reality is that creating a production-ready product that can be trusted is much more challenging for several reasons.
Lack of Domain-Specific Information
While GenAI models can produce impressive outputs, they always lack agency-specific information. Generic models typically struggle to grasp the nuances and intricacies of specific industries or subject matters. This limitation becomes apparent when the system is required to generate contextually relevant content or provide insight in specialized fields. Building a GenAI solution tailored to a specific domain demands that subject matter experts spend months inputting and labeling a huge amount of data and retraining the models, which is also expensive.
Hallucinations
GenAI systems, including LLMs, are susceptible to hallucinations. These are instances where the AI generates outputs that are not grounded in reality or are factually incorrect. These hallucinations can have serious consequences, especially when the AI is used in critical decision-making processes. While the initial 60-70% of the solution works well, eliminating the final 30-40% of hallucinations and other issues requires employing advanced techniques, including refining training data, enhancing prompt engineering, and rearchitecting the overall platform, all of which are typically costly and time-consuming for agencies.
Lack of Explainability
Another critical pitfall is the inherent lack of explainability in most GenAI solutions. These models operate as black boxes, so understanding how and why they produce a specific output can be challenging. This lack of transparency raises concerns, particularly for agencies where accountability, interpretability, and adherence to regulations are paramount. Without clear explanations for the AI’s decisions, it becomes difficult to diagnose and correct errors, leading to potential risks and lost public trust. Making matters worse, solving this issue requires teams with expert AI experience.
Cost Considerations
The cost of building and maintaining a GenAI solution can be significantly underestimated. While the initial setup might seem manageable, ongoing expenses associated with data storage, model training, infrastructure maintenance, and time spent bridging that final 30-40% can quickly escalate. Moreover, the need for continuous monitoring and updates to keep the system relevant and effective adds to the overall cost. Agencies embarking on the journey of building their own generative AI solution must carefully consider the long-term financial implications.
While the journey of building a GenAI solution may begin with a straightforward assembly of various components, the road ahead is fraught with challenges. The initial success can be deceptive, masking issues such as the lack of domain-specific information, hallucinations, and the absence of explainability. Additionally, the financial commitment required for sustainable development and maintenance should not be underestimated. As the field of GenAI continues to evolve, understanding and mitigating these pitfalls is essential for creating reliable, effective, and responsible AI solutions.
Ryan Welsh is the founder and CEO of Kyndi, a global provider of the Kyndi Platform for the Natural-Language-Enabled Enterprise, an AI-powered platform that empowers people to do their most meaningful work. To learn more visit https://kyndi.com/ or follow them on LinkedIn and Twitter.
Leave a Reply
You must be logged in to post a comment.